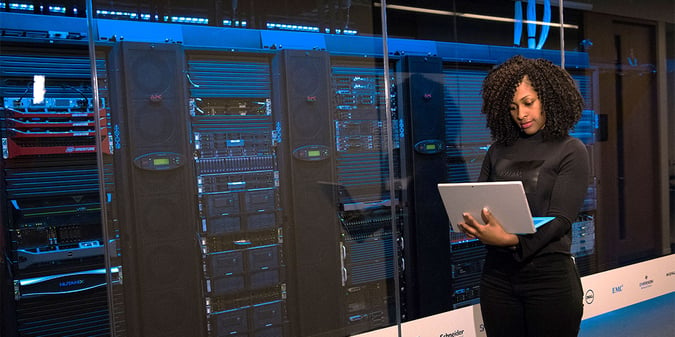
We’ve all heard of IBM’s Watson. Whether winning at Jeopardy or diagnosing medical conditions, it shows great potential and already has proven itself as a partner for humans. Honestly, I believe that it’ll do nothing but grow and develop as a learning machine. Machine-based learning in special libraries is an interesting area to explore.
Perhaps it’s overreaching to describe machines as having the ability to learn. Maybe.
There are many definitions of ‘learning’. This one echoes in my cerebrum:
“Learning is the relatively permanent change in a person’s knowledge or behavior due to experience. This definition has three components: 1) the duration of the change is long-term rather than short-term; 2) the locus of the change is the content and structure of knowledge in memory or the behavior of the learner; 3) the cause of the change is the learner’s experience in the environment rather than fatigue, motivation, drugs, physical condition or physiologic intervention.”
–From Learning in Encyclopedia of Educational Research, Richard E. Mayer
Can we change ‘person’ to ‘machine’ and still get a good definition? Maybe.
On the other hand, I worry that learning comes from failure—machines can be good at that! But the most important learning in my life hasn’t been facet and business or professional processes. It has involved emotion, empathy, sympathy and understanding. Is this machine-learning world capable of evolving beyond the scarier scenarios we read in science fiction?
Machine-based Learning in Special Libraries
“Machine learning is a method of data analysis that automates analytical model building. It is a branch of artificial intelligence based on the idea that systems can learn from data, identify patterns and make decisions with minimal human intervention.” (Source: SAS )
Check out this interesting article: Machine learning in libraries: profiling research projects rather than people
I see potential for using machine-based learning in special libraries and intranet spaces. We have a data set and profiles that are confined to the subjects and domains of interest to our clients. Whether that’s a specific field of medicine or reputational searches through the web, we have basic, definable core issues we search and provide. Can we use machine learning to harvest information from our databases and intranets and learn about our users’ behaviours—or indeed, predict them?
If libraries were to avoid keeping a record of patron activities, it would be much more difficult to build patron profiles. However, libraries do need to track some basic information, such as searches, and can offer better services to patrons if they track and analyze how resources are being used. In addition, they may be obligated to record information about access to electronic resources. There are also the benefits of machine-learning, and while “opt-in” or “opt-out” models can limit profiling, many machine-based learning algorithms will only work well if most people participate. We can work within anonymized and aggregated data guidelines and policies to achieve these goals.
While I am not a fan of over-regulating and tying down new technologies while they’re in the infant and toddler phases of development, I do believe that librarians are excellent people to review and comment on the ethics (and the potential) of these learning initiatives. Indeed, recently, the Toronto Board of Trade recommended that the Toronto Public Library was the top, most trusted place to house the Sidewalks Labs data. Sidewalk Labs is Google’s initiative to build a Sidewalk Toronto—a place where SmartCity little and big data can be collected and purposed for good. It’s an excellent test bed, but full of red flags to be addressed by experimenting. Whom do you trust?
-Stephen
Stephen Abram is a popular Lucidea Webinars presenter and consultant. He is the past president of SLA, and the Canadian and Ontario Library Associations. He is the CEO of Lighthouse Consulting and the executive director of the Federation of Ontario Public Libraries. He also blogs personally at Stephen’s Lighthouse. Check out his book from Lucidea Press, Succeeding in the World of Special Librarianship!